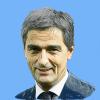
The European Data Protection Supervisor has invited three staff members who have recently joined the institution to share their own thoughts on various topics of interest. In this way, the European Data Protection Supervisor wishes to stimulate the exchange of ideas and perspectives in the field of data protection and beyond.
Brain Plugs and the Evolution of Privacy – by Leon Rossmaier
About two weeks ago, a Silicon Valley company specialised in brain-machine interfaces came up with a solution to implant data-threads of microscopic width into the human brain. Of course, other attempts to implant such brain-machine-interfaces already exist. The procedure used in this particular case comes with more bandwidth, flexible and less damaging plugs, and is executed by a skull-drilling robot that promises to make the whole operation cost efficient and ready for the consumer market in no time. The first human patient will receive the implant about one year from now. Of course, this technology is supposed to help people with neural damage, but as things go, it might be available to everyone in the not-so-distant future.
In addition to the questions these developments raise about the human condition or the next step in human evolution - as transhumanists call it, there are also interesting implications for privacy. It is clear that technology like this needs the necessary attention that protects us from possible Black Mirror style dystopias. One way to approach this challenge is to think about privacy in that context. Following the three most prominent conceptions of privacy, I ask the question of how the conception of privacy could answer to these recent technical developments.
The concept of privacy comes from ancient Greek philosophy. Aristotle distinguished between the public space and the private. The public refers to the space where citizens participate in the political life of their cities and where the necessary interactions with their fellow citizens take place. The private, on the other hand, describes the intimate space shared with family and friends, where people generally retreat from political life. This is a negative notion of privacy, not telling us what privacy is, but rather what it is not. It is a conception that implies retreating, or hiding from, a certain kind of exposure – the public.
Today’s debates around digitalisation and digital technologies have made privacy a significant topic of interest. This shows us a second definition of privacy. The notion of privacy that is discussed in these debates differs from the Aristotelian account and usually refers to privacy as a right, putting consumer protection front and centre. Privacy in this sense is seen as a right to execute a sort of control over one’s own information. Regulations like the GDPR reflect this notion of privacy, aiming to give back control to the user - in this case the data subject. The principles of informed consent or the right to be forgotten are just two of many examples of this.
As the example of brain-machine interfaces illustrates, the relationship between us and the technology we create is becoming increasingly intertwined. We do not use technology externally anymore, instead we are starting to implement it into our bodies, and even into our brains. This is creating a society that will rely and depend on technology to a historically unique extent. Sociologists nowadays often refer to socio-technical-systems when speaking about the interplay between humans and technology. This marks a shift from a dualistic paradigm of the relationship between society and technology to a rather holistic one that reflects this complexity more appropriately.
Of course, information processing digital technology is the most relevant kind of technology when discussing privacy. In the ever more complicated relationship between humans and technology, the question arises as to whether executed control over information can really be a meaningful way to protect people’s privacy. Reading the privacy agreement when signing up for an online service is already a cumbersome task. How would instruments like informed consent work in a world where we are even more connected, produce even more data and even become part of the internet of things ourselves?
The third prominent way to think about privacy argues that the exchange of information is a vital part for social life and that we should not be the ones to decide in every single situation if we consent to a ten-page privacy agreement or not. In the end, we do not value control, we value appropriate social interaction. The theory behind this is called contextual integrity. Explained in a few words this means that our expectations on whether and how our information is shared strongly depends on the social context. If we tell a secret to a friend, for example we expect her not to share this information. If we visit our physician on the other hand, it might be useful if he shared our diagnosis with a specialist who can help us find the best treatment. In every social context there are entrenched norms that help us determine whether the sharing of our information is in our own interest or not. Privacy in this sense is the appropriate flow of information in a social context, according to those norms, appropriate meaning in our own interest.
In a society that faces an ever-increasing convergence between technology and social life, as well as more and more digital solutions, the approach of contextual integrity would force policymakers to pay more attention to people’s contextual expectations rather than providing them with tools of control that some may regard as being inconvenient for everyday life. Privacy would then be measured based on people’s interests, according to the roles they fulfil in certain social situations.
When it comes to our fundamental values like human dignity or freedom of expression we could simply state that they are entrenched norms in all social contexts. Applied to the context of the aforementioned brain-machine interfaces this would imply that for example the norm of freedom of choice must never be violated. In addition to that, people might love to have the recent update on the device that stimulates their brain in a way so they can move their arm again after having had a stroke. Their consent is not actually needed as long as the update is coherent with their interest of getting this ability restored.
This allows us to extend our current notion of privacy as a form of control to privacy as the appropriate flow of information in our own interest. Of course, fundamental rights and values are and should always be in our interest. The practices of today’s multinational companies have not been stopped by handing control back to the consumers. We should therefore ask ourselves what really is important to us. After all, it is not control per se that we value. It is the social interactions and the appropriate consideration of our interest.
On privacy and algorithmic fairness of machine learning and artificial intelligence – by Lukasz Olejnik
When big chunks of user data collected on an industrial scale continue to induce constant privacy concerns, the need to seriously address problems of privacy and data protection with respect to data processing is important as never before. Data is increasingly fed into machine learning models (i.e. “artificial intelligence” facilitating automatic decision making), potentially raising many concerns, including whether decisions made by such models are fair for users. Indeed, research not only indicates that machine learning models may leak the learned user data, including personal data. Concerns over biased outputs and fairness (how I view “fairness” is discussed below) are also increasingly apparent and without doubt they contribute to the mounting concerns over potential discrimination risks.
The pace of adoption of deep learning-based methods may also soon highlight the relation between privacy and data protection on the one hand, and fairness on the other hand - thanks to the rising popularity of differential privacy (originally introduced in this seminal work). Adopted for specific purposes by some of the biggest companies, the technique became widely recognised in the industry. In response to identified issues, such as the risk of reconstructing user profiles, US Census Bureau adopted it for the 2020 survey. Indeed, when applied to the right problem, the technique can provide benefits.
Differential privacy was also discussed during the EDPS’ 2019 Annual Internet Privacy Engineering Network (IPEN) workshop, which focused on what is ‘state of the art’ technology in data protection by design; privacy engineering, anonymization and pseudonymisation among the mentioned core topics. This technique can be helpful in privacy engineering. But what is the nature of the method?
Differential privacy is actually not a one-size-fits-all method; “Differential privacy is not a product”. Rather, differential privacy is a statistical property that methods such as algorithms or protocols can meet. Satisfying the property ensures that data is processed in a way that puts stringent limits on the risk of data leakage. A process (i.e. algorithm) is differentially private when its result (e.g. computation) is indistinguishable when applied to input data. Differential privacy wants to avoid a situation when an adversary wants to draw conclusions about user’s personal data based on the outcome of the algorithm. If differential privacy is used properly, this will not be possible. Differential privacy is a very strong method of privacy protection. It is the only existing model where privacy guarantees can be proven with mathematical precision. To put it simple, the technique offers learning useful information without holding data relating to individual users, and in a way that inferring data about individuals may be next to impossible.
To achieve the constraints of differential privacy, carefully tuned noise is added in the computation of the result, in a way to meet the guarantees but preserving the data utility. This means that the algorithm outcome is not exactly precise. Assuming the application to the right problem and having a big enough dataset, the actual results can be “good enough” in practice. Differential privacy is therefore about the trade-off between accuracy (data utility) and privacy (i.e. risk of inference about users). Imagine a simple example of an algorithm providing data about the number of users with a specific (e.g. income, gender, preferred emoji). For a more direct example, let’s say we publish statistics about the number of people at EDPS that like cheese and this number is 39. Let’s say that an employee joins EDPS, and this statistics is then updated to 40. It becomes very simple to conclude that the employee in fact does like cheese. Differentially private computation would however not output 39 in the first case and 40 in the second, but different numbers that would include mathematically carefully tuned random noise; where for example the differentially private count could be 37 prior the joining of the new person, and 42 afterwards; making it impossible to conclude that any particular individual at EDPS likes cheese - so although I like cheese, my individual contribution to this statistics is well hidden. Practical applications can be much more complex, of course.
Differential privacy finds its use in many applications, it can also be applied to provide privacy guarantees of user data that is collected to train deep learning models (a subset of machine learning), giving rise to privacy preserving learning. Privacy in machine learning is important, for example in light of research works demonstrating the ability of recovering images from facial recognition systems, or even personal data such as social security numbers from the trained models. But focus put just on security, privacy and accuracy might not be enough.
It is increasingly apparent that biased data used in machine learning model training may become reflected and even reinforced in the predictive output, making such biased input data influencing future decisions. This problem is especially acute when such machine learning applications would result in unfair decisions for selected small subpopulations in otherwise larger datasets. In doing so, algorithms would lead to the optimisation of answers towards the larger, better represented groups. To put that in a practical context, instead of considering the all too academic notion of a “small subpopulation”, think about the actual traits such as gender, medical conditions, disability, or others. A generalising algorithm might inadvertently be made to “prefer” the better represented data records. Those concerns are important from the digital ethics point of view, making issue of fairness an area of focus.
But what is fairness in the first place? In this post, I do not consider fairness in the meaning of Article 8 of the Charter of Fundamental Rights or Article 5(1)(a) GDPR. Rather, I use fairness as a technical term. Machine learning is a mathematical concept so notion of fairness must be designated within this realm. Many mathematical metrics of fairness exist but discussing this fascinating area is outside the scope of this post - let’s just say that there is no standardised “ideal” metric of fairness. But a particular one to consider could be the equal opportunity, also known as equality of true positives. In this metric, fairness is about not conditioning of decision outcomes on specific, perhaps hidden traits, in a way that those protected, underrepresented groups are not treated unfavourably. In practice, one could imagine these groups being described with a demographic character, based on traits such as gender, age, disability or so on. A concrete example situation could be in hiring decisions - the chances of being hired should be equal, regardless of attributes such as gender or age.
When having a direct access to the data, methods to compensate for model unfairness can be devised and applied. But what if one actually does not have access to data, like in the case of differentially private methods? The matter may become complicated.
But it turns out that in reality the matter is actually much more complicated, as pointed out by latest research highlighting an inherent relationship between privacy and fairness. In fact, it becomes apparent that guaranteeing fairness under differentially private AI model training is impossible when one wants to maintain high accuracy. Such incompatibility of data privacy and fairness would have significant consequences. With respect to the potential of unfairness of some of the standard deep learning models, when it comes to fairness, the current differentially private learning methods fare even worse, reinforcing the biases and being even less fair to a great degree. Results like that should not exactly come as a surprise to implementers and deployers of the technology. Hiding data of small groups is actually among the features of differential privacy. In other words, it is not a bug but a feature of differential privacy. However, this feature leading to decrease of precision might not be something desirable in all use cases.
The problem is fortunately gaining traction. One may expect that further work will not only shed more light on the potential consequences and the possible impact on fairness. There are already works exploring the possible trade-offs even further. Such as the work published in June 2019 (Differentially Private Fair Learning) which further explores these trade-offs between privacy and fairness and introduces methods addressing some of the issues, even though for a price - and that means having access to some of the sensitive traits of those potentially affected small subgroups. But if those traits are truly sensitive (i.e. special category of data) this would mean that the system in question would actually process this data.
As with any system designed to be used for special cases, all the trade-offs need to be carefully considered. Indeed, to deploy a differentially private system today, considering the use on a case by case basis might be the way to follow anyway, since differential privacy is not a technique for universal use (it’s not a magical solution to all the problems). When analysing the use case, experts and deployers should be wary of the full consequences for the fundamental rights and freedoms with respect to personal data processing.
Specifically we could envision:
- Deployers considering problems holistically, understanding their requirements, as well as the needs of users. Considering the latest state of the art when improving privacy protection is already an essential privacy by design need. While we do not know what might be the potential undesirable impact of already deployed differentially private methods, if any, it may be a good idea to include considerations such as fairness in the risk assessment when considering the impact on fundamental rights and freedoms.
- Indeed in some cases, for example when processing large datasets or applying artificial intelligence methods, in which the innovative use of differential privacy may be useful may already warrant carrying a data protection impact assessment (DPIA) may have been a beneficial or needed step anyway. In such cases, DPIA would currently be the right place to include such a detailed technical analysis, to explain the rationale behind the chosen methods and their configurations (including the configuration parameters).
- When designing AI systems, assessing and understanding of the algorithmic impact should be seen as beneficial; DPIA should serve as a good place for such considerations.
To summarise, when considering any complex system many aspects need to be weighed in. This analysis is often done on a case by case basis. Differential privacy can help in the development of systems that provide trade-offs between privacy and data utility, with provable guarantees. But some settings, such as in the training of neural networks with, the use of differential privacy or not, considering other aspects may be also of note. Exacerbating unfair treatment of disadvantaged groups should not become a feature of modern technology. Fortunately, today these challenges start to be explored. In case of the potential implications of differentially private deep learning on fairness the most important piece of initial work is ongoing: potential problems are identified and now work should continue to address it. In the meantime, big deployers of differentially private methods may consider it justified to explain if the specific ways in which they use the technology might have undesirable impact, if any at all. That would simply be a matter of transparency.
New exciting technologies can help process data with respect for privacy. Differential privacy offers significant improvement for privacy and data protection. Still a nascent technology, and by this very nature its use warrants case-by-case analyses. This is fortunate because organisations or institutions considering to use differential privacy have the opportunity to see the big picture and address their actual, specific situation and needs. Improving privacy and data protection of data processing with new technologies and conforming to the latest state of the art should be the standard. But it’s worth to keep in mind of what may be the overall implications. Where needed, explaining these technical considerations and rationale in a document like data protection impact assessment might be helpful.
Generation Z and Fake News – by William Sharpe
With the election of Donald Trump in 2016, and the Brexit Referendum result of the same year, the controversial notion of ‘Fake News’ has taken a somewhat centre-stage position within the Media and global Politics. But what is ‘fake news’? Is it just opinions we don’t agree with? Or is it the product of a modern world where the line between truth and fiction is becoming increasingly blurred? And how do we guard against its effects in this technological World?
As the name suggests, ‘Fake News’ is best defined as news that has been falsely constructed, usually in order to push an agenda on someone or a group of people. One example of this is the infamous Brexit bus, with its massive statement in even bigger letters; “We send the EU £350 million a week, lets fund our NHS instead.” As most of us will know, this figure was admitted to be exaggerated the morning after the Brexit vote. Since the Referendum result only gave a victory by 4%, it is not unreasonable to assume that this lie may be responsible for at least some of the votes which decided the outcome of the referendum. Whether you agree with Brexit or not, this shows what an impact political lies can have on people’s opinions and the political direction of any country. Despite this, the constructors of this falsehood are still big names within UK politics, perhaps the biggest. No real consequences were felt, and this only scratches the surface of the misinformation spread throughout the World on a daily basis.
Generation Z and the ‘Millennials’ are perceived to be the generations of technology. We are the ones who grew up online, interacting with each other through applications and websites such as Snapchat, Instagram and Facebook. Our lives and viewpoints are broadcast for everyone to see, including those hoping to control them. Because of this, corporations such as Facebook can easily figure out which ‘News’ stories will strike a chord with us as individuals, from cute animals to political scandals. However, since social media is not an actual news outlet, they have no responsibility to fact check any of the stories that appear on their websites. This can lead to falsehoods, which are often detrimental to society, being spread around the World in a matter of minutes. Since our personal data is collected from an early age, these generations may be more susceptible to fake news than any other generation before.
Much like targeted advertising, corporations such as Facebook use our personal data to target News stories and articles to us. These can be from incredibly unreliable sources, riddled with misinformation and misguided opinions. In order to help me explore this subject, I conducted a survey with a sample of 46 teenagers (aged 16-18) from the South-East of England, with a range of political ideologies and cultural backgrounds. Of those surveyed, 61% said they used social media as a News outlet, and around 80% agreed that the Internet made them more susceptible to the effects of Fake News. Data is power in this modern world, and since our data has been held online from an early age, the amount of power that these corporations have over us is unimaginable. Personal data is used to drip-feed us with fake news and political biases that they know will resonate with us and keep us in our political bubbles. The Internet is full of opinions, but websites like Facebook will make sure we are mainly confronted with ones we agree with, whether they are based on facts or not.
So what sources can we trust? Who should we turn to for accurate and reliable news? 70% of my survey respondents view BBC News as at least “quite trustworthy”, and while News Corporations like this one are generally more reliable, we must remember that a degree of political bias will still be present in these news articles, despite their efforts to remain neutral. However, only 54% of survey respondents say they use BBC news as one of their main sources of information, while 61% use Instagram and/or Snapchat for the same effect. These are worrying numbers, as social media websites such as these ones are rampant with falsehoods and forced political agendas. However, before we solely blame Social Media for the spread of fake news, we must remember where news used to come from; newspapers. In my survey, roughly 80% of respondents said tabloids (eg. The Sun & The Daily Mail) are untrustworthy, an opinion which is shared by many across the World. Before Social Media, fake news was mostly spread through the tabloids. However, the effects felt by these lies were minimised as they only affected readers of those particular newspapers, whereas Social Media influences the opinions of most of the population, whether they like it or not. Perhaps this is why older generations were less susceptible to the effects of ‘Fake News’ than modern generations.
The best thing my generation can do in a world of misinformation is to fact-check our sources. Instead of choosing our news sources based on ‘Convenience’ (which 74% said they do), we should make sure that the news we are receiving is both accurate and reliable. However, we are constantly drawn to convenience, and since we already use Social Media on a daily basis, it is unrealistic to expect everyone to ignore news articles they see on applications such as Facebook and Instagram, especially since they are specifically targeted to us based on our personal data. The technological age has brought a rich reservoir of information to our fingertips, but it has also allowed the spread of misinformation to accelerate and reach a larger proportion of the population. This is a scary prospect, and it is hard to suggest a suitable solution to this problem. If corporations such as Facebook are told to stop the spread of misinformation on their platforms, they themselves may enforce a political bias on the population, and why should it be up to private firms to decide what is right and what is wrong? Even the Government deciding what is true and what is false may be problematic as these terms are largely subjective, especially in the Modern World filled with different opinions and interpretations. Therefore, it must be left to the individual to make sure the ‘news’ we are sharing is as accurate and free from bias as possible.